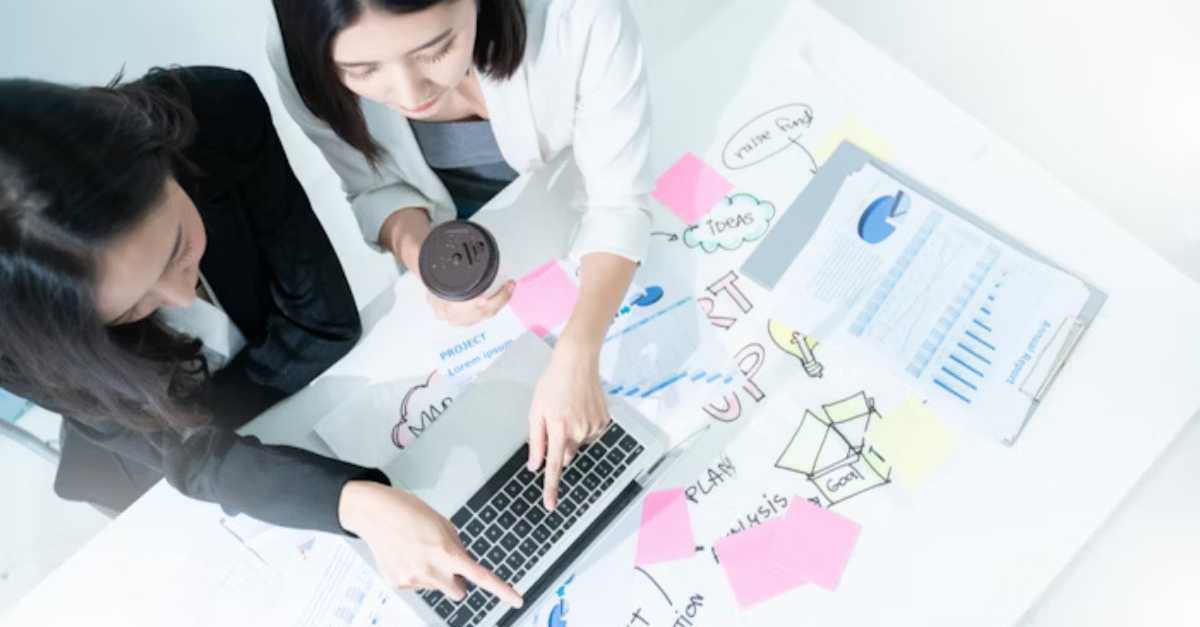
Can Meridian EDMS be Used as an Enterprise Content Management (ECM) Tool?
By design, Meridian is an engineering document management system (EDMS). But once in use by Engineering, the tool can be extended to support more ...
Solutions
Workplace Management Solutions
Real Estate Management Solutions
Maintenance Management Solutions
Energy Management Solutions
Engineering Document Management Solutions
Asset Management Solutions
Automate campus scheduling for classes, meetings, and exams with our EMS software.
Plan and manage conferences effortlessly with EMS software to impress guests and streamline operations.
Boost workplace flexibility and maximize space use with seamless desk and room booking.
Organize workplace or campus events smoothly, creating memorable experiences.
Optimize workspace, manage allocations efficiently, and reduce costs with our space management solutions.
Deliver projects on time and within budget by improving communication, collaboration, and efficiency with our software.
Streamline lease accounting for ASC 842, IFRS, and GASB compliance.
Manage leases efficiently by tracking key dates, analyzing costs, and ensuring compliance.
Centralize data and analytics for better insights, faster negotiations, and revenue growth.
Centralize facility and asset maintenance, automate work orders, and ensure compliance with our CMMS software.
Extend asset life, reduce downtime, and prevent costly repairs with data-driven monitoring.
Prevent equipment failures and extend asset life by detecting and addressing issues early.
Make sustainable, cost-efficient energy decisions by monitoring and optimizing power consumption.
Remotely monitor and control equipment with real-time data to predict issues, boost efficiency, and reduce downtime.
Easily share and collaborate on documents, creating a single source of truth for engineers and contractors.
Manage and analyze assets across their lifecycle to schedule maintenance, reduce downtime, and extend lifespan.
Improve visibility, automate work orders, and ensure compliance for efficient facility and asset management.
Resources
Browse our full library of resources all in one place, including webinars, whitepapers, podcast episodes, and more.
Support
Looking for access to technical support, best practices, helpful videos, or training tools? You’ve come to the right place.
About Accruent
Get the latest information on Accruent, our solutions, events, and the company at large.
Asset tagging in your ALIM can now be automated using the power of machine learning. See how it works and its many benefits, including increased efficiency.
Have you ever struggled to find the manual to put a piece of furniture together or repair a coffee machine? How about searching for the right documents during tax season? It can be frustrating – and not finding the information you need can lead to broken items, snowballing costs and plummeting efficiency.
If you work in manufacturing, you know that this frustration is multiplied by about a million on the plant floor. And the potential costs of not knowing what documents go with which assets – or which documents accurately reflect the as-built environment – can be much higher, leading to lower efficiency, siloed teams, slow operations and more.
Automating your asset tagging and referencing can help resolve these concerns.
If you want to digitally transform or automate your operations, you need to be able to tell your Asset Information Management system which assets are referenced by which documents -- and vice versa. This will not only allow your team to easily find the documents they need to do their job, but also ensure that your documentation is always up-to-date and that you have the information you need at hand to overcome pressing manufacturing challenges.
Until now, this hasn’t happened – and process engineers, document controllers and maintenance technicians have been the human interface to connect the assets (facilities, functional locations, equipment, pipes & lines) to documents (layout drawings, process and instrumentation diagrams, operating procedures, lockout tagout procedures). This has required extensive manual work – and it’s been more inefficient than it should be.
With the right tools, though, you can automate this process for easy referencing and increased efficiency.
With the availability of digital information— and accurate representations of facilities, utilities and other industrial assets— it is now possible to automate asset tagging and referencing multiple assets to multiple documents and vice versa. To enable this capability, you simply need to teach your Asset Information Management (AIM) system how to recognize asset tags in technical documents and match those tags to functional locations or physical equipment.
An obvious location to start looking for asset tags is on Process and Instrumentation Diagrams or P&ID's. P&ID's provide a schematic overview of the production process with an overview of pipes, lines, equipment and instruments. The items on the P&ID are usually tagged according to International P&ID Standards i.e., ISA-5.1, DIN 19227, PIP PIC001, ISO 14617 or BS 1646. These standards allow the machine or system to learn and recognize symbols that are used on a P&ID like:
Based on this classification information – in combination with a naming convention and sequence number— the system can learn how to recognize asset tags.
How exactly is this accomplished? By utilizing machine learning capabilities within your Asset Information Management system.
The first step in the process is to teach the system how to analyze the text describing asset tags based on the naming conventions. Keep in mind that there can be multiple naming conventions for a single tag type. For example, you can tag for usage and/or location of a particular asset. To recognize all tags, the system uses an algorithm with multiple patterns to recognize them. Also '*' operators and concatenation of multiple attributes can be applied in the algorithm to improve the recognition of asset tags.
The second step is to identify the asset tag in the multiclass classification and to create a relationship between the asset tag and originating document. When the asset tag is found, the link can be made. When the asset tag is not available or not found, the asset tag can be created or marked as unavailable. It is also possible that the algorithm will need to be optimized to further improve the matching criteria.
The third step is to apply 'hotspots' to the document where the asset tags are found. 'Hotspots' are image classifications in a document to identify asset tags. When a hotspot is selected, the user can navigate to the asset tag to find more information on the asset.
By following these steps, users will be able to find asset tags in documents, highlight the hotspots in P&ID's, view the asset tag details and navigate to the asset tag to find other related documents.
There are multiple benefits of machine learning for asset tagging, including:
For more information -- or if you want to learn more about machine learning, asset tagging and hotspots – you can contact me at coen.vromans@accruent.com.
By design, Meridian is an engineering document management system (EDMS). But once in use by Engineering, the tool can be extended to support more ...
Explore why “free” document management tools are actually costing your company time and money, and how to solve for it by switching to Meridian EDMS.
Discover four ways Meridian EDMS’s document version control functionality can increase collaboration while decreasing project turnaround time.
Subscribe to stay up to date with our latest news, resources and best practices