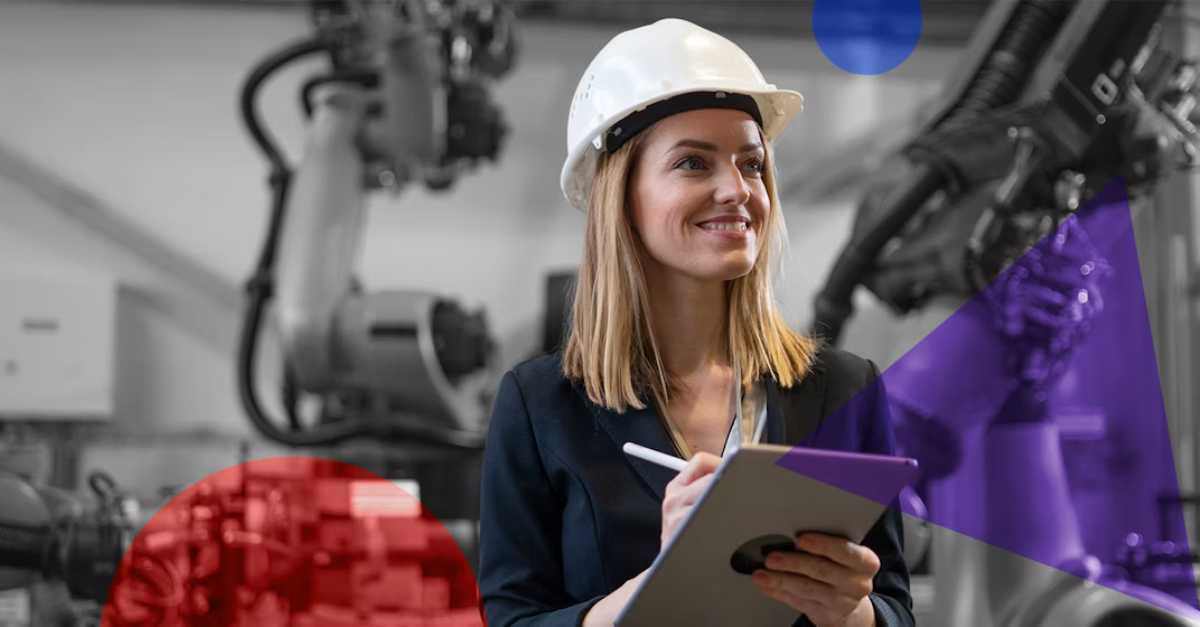
What is IoT remote monitoring?
IoT remote monitoring helps companies manage their assets remotely, in real-time, with devices and sensors that collect data and transmit it over the ...
Solutions
Workplace Management Solutions
Real Estate Management Solutions
Maintenance Management Solutions
Energy Management Solutions
Engineering Document Management Solutions
Asset Management Solutions
Automate campus scheduling for classes, meetings, and exams with our EMS software.
Plan and manage conferences effortlessly with EMS software to impress guests and streamline operations.
Boost workplace flexibility and maximize space use with seamless desk and room booking.
Organize workplace or campus events smoothly, creating memorable experiences.
Optimize workspace, manage allocations efficiently, and reduce costs with our space management solutions.
Deliver projects on time and within budget by improving communication, collaboration, and efficiency with our software.
Streamline lease accounting for ASC 842, IFRS, and GASB compliance.
Manage leases efficiently by tracking key dates, analyzing costs, and ensuring compliance.
Centralize data and analytics for better insights, faster negotiations, and revenue growth.
Centralize facility and asset maintenance, automate work orders, and ensure compliance with our CMMS software.
Extend asset life, reduce downtime, and prevent costly repairs with data-driven monitoring.
Prevent equipment failures and extend asset life by detecting and addressing issues early.
Make sustainable, cost-efficient energy decisions by monitoring and optimizing power consumption.
Remotely monitor and control equipment with real-time data to predict issues, boost efficiency, and reduce downtime.
Easily share and collaborate on documents, creating a single source of truth for engineers and contractors.
Manage and analyze assets across their lifecycle to schedule maintenance, reduce downtime, and extend lifespan.
Improve visibility, automate work orders, and ensure compliance for efficient facility and asset management.
Resources
Browse our full library of resources all in one place, including webinars, whitepapers, podcast episodes, and more.
Support
Looking for access to technical support, best practices, helpful videos, or training tools? You’ve come to the right place.
About Accruent
Get the latest information on Accruent, our solutions, events, and the company at large.
Understand the complexities of artificial intelligence and the keys to effectively integrating it into your organization.
Artificial intelligence (AI), the Fourth Industrial Revolution (4IR), a more predictive environment. These aren’t just buzzwords, but key technological advancements and features on everyone’s wish list as companies fight to digitize and modernize their organizations.
And with good reason – a truly predictive environment can transform a manufacturing organization, unlocking intelligence that can be used to streamline processes, enhance the technician experience, reduce waste and maximize efficiency. What’s more, with a bevvy of sensors and networks across the operating environment, manufacturing organizations are particularly well-suited to this kind of transformation.
But creating such an environment is no easy task, and AI isn’t a magical technology that you can buy and suddenly achieve a digital environment. On the contrary, AI is only as strong as the data that informs it and the team that uses it. This is something that a lot of businesses don’t consider, and something that must be acknowledged to succeed.
Artificial intelligence (AI), as the name implies, refers to any machine that displays intelligence. AI-capable machines, by extension, are those that can perform tasks that would have previously been thought to require human intelligence.
And we see AI in action every day: voice recognition, face recognition, natural language processing, smart automations, complex analytics and predictions – these are all examples of AI.
In manufacturing, in particular, it’s clear that AI technologies can be a game-changer at every level of the value chain, leading to measurable benefits like:
All of this, in turn, can translate to big money. In fact, a 2017 PwC analysis showed that global economic output, as measured by gross domestic product (GDP), could be 14% higher in 2030 than baseline projections of $114 trillion, as a result of the expected growth of AI. This translates to an additional $15.7 trillion.
And the big players are recognizing this potential. At the 2019 Global Manufacturing and Industrialization Summit (GMIS) in Russia, participating experts stressed how the manufacturing sector could harness AI and Fourth Industrial Revolution (4IR) technology to drive development and shape the industry over the next several years. The overarching message? Get on board or get left behind.
For this reason, many manufacturing plants are trying to incorporate AI and IoT into their organizations in some capacity – and the most forward-thinking and well-funded are working to use this tech to create a more predictive environment.
And in the wake of COVID-19, this has become a have-to-have as organizations aim to thrive in a multi-site, remote environment.
So, what does AI in manufacturing look like? Well, it generally centers around:
And these technologies can be incorporated into all stages of manufacturing operations to enhance the technical experience, allow for broader insight and to streamline operations. Specifically, AI can be used in:
What’s more, these strategies are proven to work. That’s why 25% of companies in the US and 26% of companies in the UK are incorporating AI – and the rest of the world is sure to follow.
But it’s not easy – and AI is certainly not a magic wand. On the contrary, AI implementation is time-consuming, complex and costly, and getting it right requires a comprehensive, systematic strategy. Here’s how to get it right.
To successfully implement AI, you need to have the right foundation – otherwise, you’ll face frustrated team members, low ROI and a failed implementation. Your foundation should include:
AI is not a cure-all or a set-it-and-forget-it thing. That’s because, at its most basic, AI can’t even tell you what’s going on without proper guidance. It just sees patterns based on the algorithms you set – so the most important first step is to have strong data to inform that AI and allow it to show helpful patterns. This requires reliable data acquisition, management and governance.
In the manufacturing context, this usually starts with sensor data collected from equipment on the factory floor. And the sheer quantity of data can be overwhelming, which is why many organizations are building “data lakes” to house their raw data.
Importantly, though, it’s not just about collecting all the data you’ll need. It’s also about organizing it and effectively mapping your data objects, defining your metrics, monitoring your performance data and creating an overarching data management system.
In short, your data management should include:
In one survey about AI and barriers to implementation, 22% of respondents noted that organizational culture was a barrier, and 16% cited concerns about job security as a concern.
Without the right company culture and high adoption of your AI tools, any digitization effort you make is destined to fail. To this end, there are a couple of important things to keep in mind here:
PwC puts a company’s digital maturity into four categories: digital novices, digital followers, digital innovators and digital champions.
What’s more, they found that 69% of digital champions have implemented, piloted or plan to use AI within their business, compared to 10% of digital novices. In short, where you are on your digital journey will certainly impact what kind of AI you implement, as well as the success of your efforts.
If you find that you’re a digital novice, but you’re looking to build a more data-driven culture, you must:
And working toward this kind of culture is one of the biggest trends you’ll see in 2021 and beyond.
As we mentioned, it’s important to be clear about the type of AI you’re going for and what you want AI to accomplish in your business. Again, a fully autonomous AI environment is not feasible – or even beneficial – for all organizations. There are four types of AI to consider here:
Companies that are just starting to implement AI should start with assisted intelligence — that’s integrated with legacy IT systems and industrial IIoT — and work their way up. This will not only streamline the process but also help with bottom-to-top process overhaul while maximizing adoption.
Manufacturers should focus their efforts on the places where AI can add the most value and then drive those solutions to scale. This can help prove the ROI of AI efforts and quell any concerns with this change in tools and processes. To this end, start by:
If your team doesn’t have AI know-how backed by standardized processes, you won’t be able to implement and maintain AI solutions at scale. Ideally, your AI skills and structure should include:
For the people that aren’t data experts – but still need to use your solutions – it's important to maximize visibility, transparency and ease-of-use surrounding your AI solutions. This can not only help scale solutions but also simplify adoption efforts.
This will necessarily require that your business-critical tools, like your CMMS and EDMS systems, are connected and sharing important information. This will bring AI solutions into the day-to-day lives of your technicians, plant managers and other key employees.
Finally, you need the right systems, which should include:
Here are some use cases that show how AI can really be implemented in manufacturing.
A form of assisted intelligence, “smart” maintenance of assets is low hanging fruit when it comes to AI adoption in the manufacturing space. That said, even though it’s fairly straightforward, this kind of AI adoption can lead to many benefits, including lower downtime, lower maintenance costs and increased productivity. It can also help teams:
The power of this approach can be seen in the following example from Capgemini:
“A leading automotive manufacturer that was struggling to reduce machine stoppages and minimize production losses. It wanted to identify machines and production lines in advance where faults are likely to occur, jeopardizing sales and final deliveries to customers. With an AI-enabled predictive maintenance solution, it was able to accurately identify machines and lines that were most likely to fail and take proactive remedial action.
In a month where significant failures were anticipated, intelligent maintenance allowed 300 additional cars to be produced. This was in addition to the output that might otherwise have been lost because of downtime and maintenance.”
Similarly, analyzing process parameters can help you predict and prevent possible quality issues. This can be accomplish using on-floor high-resolution cameras, which can visually inspect the line and capture trends that you may not otherwise have noticed. This can not only increase efficiency but also cut time of in-person inspections and help with strict compliance regulations in certain industries.
A case in point is Audi, which, “installed an image recognition system based on deep learning at its Ingolstadt press shop. Several cameras installed directly in the presses capture images of pressed sheet metal. The images are then analyzed by the AI system to identify even the finest cracks on the metal sheets. The system was trained using several million test images, drawn from presses in Audi’s Ingolstadt plant and several other Volkswagen plants. This helped achieve a very high accuracy,” and address quality concerns proactively.
AI can also be used to predict changes in consumer demand which, in turn, can help organizations:
For manufacturer Danone, this kind of improved efficiency allowed them to, “meet demand from product promotions and achieve its target service levels for channel or store-level inventories. The system led to a 20% reduction in forecast error, a 30% reduction in lost sales, a 30% reduction in product obsolescence, and a 50% reduction in demand planners’ workload.”
These examples only scratch the surface of how AI can be used in a manufacturing context and the role that operational AI will play in future digital transformation efforts.
That said, one thing that is clear is that AI is quickly becoming a have-to-have in the maintenance space, particularly as companies look to scale, meet rising customer expectations and beat the competition.
Want to learn more about how to implement AI and make it a success in your business? Reach out to one of our experts today.
IoT remote monitoring helps companies manage their assets remotely, in real-time, with devices and sensors that collect data and transmit it over the ...
Discover how integrating the power of IoT remote monitoring with optimizing space usage in the workplace can lead to significant cost savings for ...
Explore the essentials of IoT remote monitoring in this introduction.
Subscribe to stay up to date with our latest news, resources and best practices