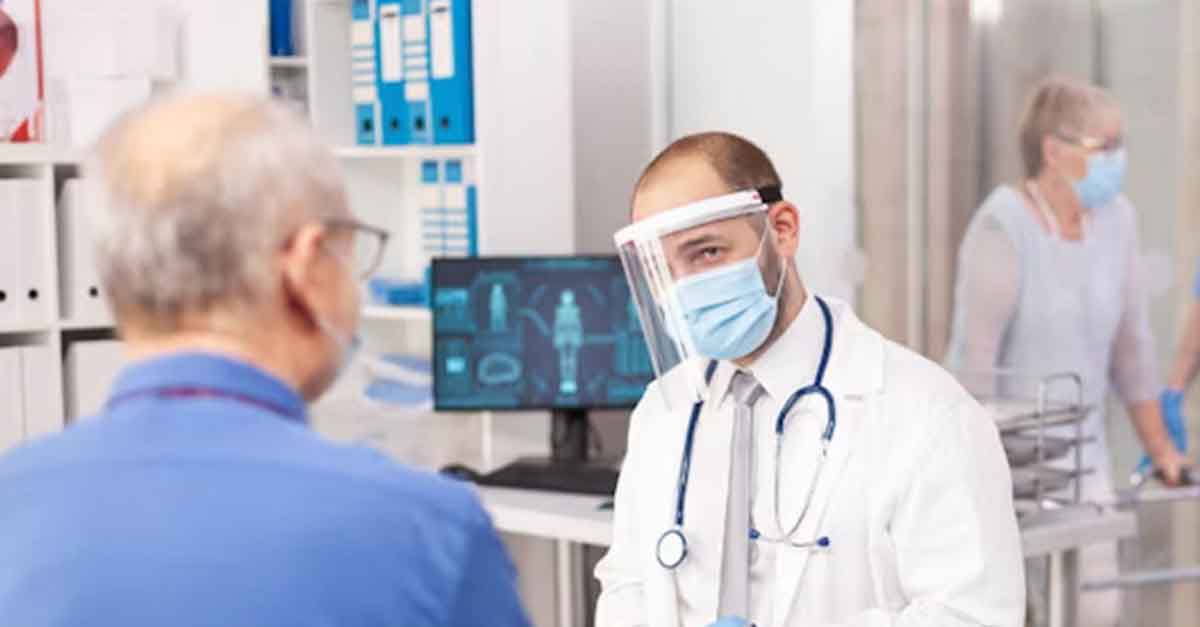
COVID-19 Impact on Hospital Operations & Readiness Needs
Discover what steps hospitals can take to handle the pandemic's many challenges, including a new COVID-19 part predictor for ventilators and ...
Solutions
Workplace Management Solutions
Real Estate Management Solutions
Maintenance Management Solutions
Energy Management Solutions
Engineering Document Management Solutions
Asset Management Solutions
Automate campus scheduling for classes, meetings, and exams with our EMS software.
Plan and manage conferences effortlessly with EMS software to impress guests and streamline operations.
Boost workplace flexibility and maximize space use with seamless desk and room booking.
Organize workplace or campus events smoothly, creating memorable experiences.
Optimize workspace, manage allocations efficiently, and reduce costs with our space management solutions.
Deliver projects on time and within budget by improving communication, collaboration, and efficiency with our software.
Streamline lease accounting for ASC 842, IFRS, and GASB compliance.
Manage leases efficiently by tracking key dates, analyzing costs, and ensuring compliance.
Centralize data and analytics for better insights, faster negotiations, and revenue growth.
Centralize facility and asset maintenance, automate work orders, and ensure compliance with our CMMS software.
Extend asset life, reduce downtime, and prevent costly repairs with data-driven monitoring.
Prevent equipment failures and extend asset life by detecting and addressing issues early.
Make sustainable, cost-efficient energy decisions by monitoring and optimizing power consumption.
Remotely monitor and control equipment with real-time data to predict issues, boost efficiency, and reduce downtime.
Easily share and collaborate on documents, creating a single source of truth for engineers and contractors.
Manage and analyze assets across their lifecycle to schedule maintenance, reduce downtime, and extend lifespan.
Improve visibility, automate work orders, and ensure compliance for efficient facility and asset management.
Resources
Browse our full library of resources all in one place, including webinars, whitepapers, podcast episodes, and more.
Support
Looking for access to technical support, best practices, helpful videos, or training tools? You’ve come to the right place.
About Accruent
Get the latest information on Accruent, our solutions, events, and the company at large.
Discover how to leverage data insights to make sound capital planning decisions for biomedical devices that will improve the efficiency of your organization.
In Season 1, Episode 8: Al Gresch, Mike Zimmer and special guest Michael Garel, Director of Big Data Strategy at Accruent, discuss game changing strategies to use data to understand equipment reliability and improve Capital Equipment Planning for Clinical Engineering Teams.
Welcome to the Healthcare Chats podcast where your hosts, Al Gresch and Mike Zimmer, will bring you insights to take your HTM and HFM from the basement to the board room. Healthcare Chats podcast starts now.
Mike Zimmer: Hi everybody, this is Mike Zimmer. And welcome to another episode of the Healthcare Chats podcast. We're joined today by Michael Garel. He's a phenomenal resource…[and] fashion icon. Tell us about what it is that you do here at Accruent, and we'll take it from there.
Michael Garel: So at Accruent, I've earned the title of Chief Comfy Sweater Officer [CCSO], thanks to Mr. Zimmer-
Mike Zimmer: You're welcome.
Michael Garel: You named me that. So I'll do that intro for you now. In reality, I'm the director of Big Data Strategy at Accruent. And my role is to take all this data that flows through our CMMS platforms and use it to build tools that can help our organizations, our customers, make better decisions.
Mike Zimmer: So you take a look at the data of all the industries that Accruent serves, but you've got a particular primary focus on healthcare. Is that correct?
Michael Garel: Correct. Our focus right now has really been around healthcare. We've got over 55% of the hospitals across the US, so we have a lot of really good data in the CMMS platform. And it's a really good industry. It's an industry that likes data, is very analytical and can use the insights that we're gleaning from the Biomedical devices that we're analyzing.
Mike Zimmer: Okay. So we'll get to the insights in just a second. But let's now talk about what is the data that you're pulling out of a CMMS solution?
Michael Garel: The data that we're pulling is from work orders and assets. So we know how often equipment breaks, why it broke, what was done to repair it, how many hours it took to repair, what materials were used. That sort of information we can pull out of the CMMS platform.
Mike Zimmer: Okay. Now how is that different from say…another source of that kind of data, like AHA [American Hospital Association]?
Michael Garel: There are other sources of information to provide insights about devices. However, we're really the only ones that have that empirical reliability data that we can use to deliver this information. There are other sources out there that do provide some reviews, but it's all qualitative and not quantitative.
Mike Zimmer: Got it. And it's based on survey data. Is that right?
Al Gresch: It's survey data. We've spoken to several Supply Chain Executives familiar with the sources that they use because I used them myself over the years. The feedback we got from them is they don't put a lot of stock in it, but they don't have anything better... Well, until we came up with this solution. It's because it's based on survey data from their customers versus real empirical work order data.
Mike Zimmer: And so you said that there's a lot of data, but you did not say there's a lot of good data. And so if I know anything about the way that healthcare organizations interact with the CMMS, it's that everybody does things a little bit differently.
Michael Garel: Sure.
Mike Zimmer: So for the folks listening who aren't super familiar with the methodologies that you employ to structure this data, take us through what you and your team have done. And from what I understand, it's absorbed the majority of the time that you've worked with the data. How do you get it to a point where you can start deriving insight from it?
Michael Garel: Sure. So we do have a lot of really good data. However, there isn’t necessarily a lot of clean data. So because we have such a large customer base, and because hospitals inherently don't necessarily enter their data consistently into the CMMS platform, we've had to apply algorithms to help try to clean that data at scale. We could sit there and try to manually clean all this data through spreadsheets, which is the way that it's done today. However, we built some algorithms based upon some machine learning, as well as natural language processing. We've used some neural networks to help us clean data. But I would be lying if I said that everything was automated. So you still have a manual process associated with a significant portion of our data. But what we've done is we've built tools to make it easier to clean data even manually as well.
Mike Zimmer: Okay. So that's when the machine assigns a confidence score to what it's attempting to categorize, and it goes to you saying, hey, how does this look?
Michael Garel: Correct. The algorithms that we built today, the automated algorithms…machine-learning can tie back to a source of truth. And so we've created a... We're using the FDA's Global ID databases [Global Unique Device Identification Database (GUDID)] as our source of truth today. And the algorithm delivers back its confidence in whether it actually matched to that source of truth. And so we're taking that information, and the ones where we see less than 90% confidence, we apply a manual process too. But like I said, our manual process is still improved because we've created tools that really make it fast.
Al Gresch: Mike, one of the examples that we use when we're talking about data integrity and the impact of machine learning, was that of a single device, a GE AMX-4 portable x-ray machine, which was represented in over 2,500 different ways if you factored all the variables of how the manufacturer name was entered and the different variations of the model, the different variations of the device category. So think about that. One single device, over 2,500 different ways to represent it. Crazy.
Mike Zimmer: Okay, so you've taken us through how you've cleaned this data. Now let's get to the fun part. What are some of the insights that you've been able to harvest?
Michael Garel: Sure.
Mike Zimmer: Looking at this dataset.
Michael Garel: Yeah, so we've created what we're calling, or what we've been told is sort of the consumer reports for biomedical devices. Ultimately what that is is we've been able to look at all the devices because we know when they fail, we can assign essentially a liability index to each and every Biomed device. So now when you're looking at devices in a category, you can compare those devices to one another based upon reliability index, for example, which takes into account things like how much labor each device has, its failure rates, its time between failures, its survival, its probability of survival over time. So that's kind of the basic.
Michael Garel: We've also been able to aggregate and mine things like failure rates. We've created time between failure numbers. We built an algorithm that looks at the probability of survival. So right now, for example, on infusion pumps, AHA says that infusion pumps should last 10 years. Well, if you're an organization that is taking that into account when you're deciding how you're going to depreciate equipment or plan for future capital, you may or may not have the money when it's time to replace that device. Or we've got some devices that your useful life is about six and a half years. Other devices, your useful life is 13 years. So in one case you might be leaving a lot of money on the table better spent somewhere else. In other cases, you're going to end up scrounging for money at the last minute and not being able to properly plan your capital. So those are just some of the metrics that we're building out.
Al Gresch: One of the interesting things that I've seen in looking at the analysis that you've been able to generate, Michael, is if you were only looking at your own data, and you're making decisions based on what you would see as an upward trend in failure rates, what we've seen with a number of devices with your data is you start seeing that that trend upwards, but then at a certain point it drops back down again. A lot of people might make a decision, well, I need to replace it here versus... All right, well if I hang on to this thing, it's going to settle out again, and I could get a number of years past what I would normally replace it at.
Michael Garel: Sure. And if you can plan for that increase in incidents either through staffing or training and then ensuring-
Mike Zimmer: Or parts purchases.
Michael Garel: That you have the parts on hand too at that point in time, it makes your organization operate a lot more efficiently. And then like you said, you don't pull the trigger on something prematurely just because you've seen an increase in failures of that device.
Al Gresch: We're trying to capture both the Supply Chain executive groups to help them make better capital equipment replacement decisions, but also the Biomed folks who've been providing recommendations on equipment for a long time, those recommendations aren't always followed. And so having this empirical data to say, look, if you pick this device, the labor and the cost that it's going to require to maintain over its lifetime is 30% higher than what we had been spending. You better give me some additional funding for my budget.
Michael Garel: It's getting past just the price tag on the device.
Mike Zimmer: Right.
Al Gresch: Exactly.
Michael Garel: And all the ancillary and peripheral costs associated with it.
Michael Garel: It's interesting because one of the other things we're looking at like you mentioned, is parts replacement over time for each device, as well as labor, as well as useful life. When you start to look at what your total cost of operation of equipment is, at times you might see that less expensive piece of equipment that you could buy today is actually going to cost you potentially over a fleet, millions more than if you had purchased the slightly more expensive unit. So if you make that investment now, you're going to significantly save over time. And that's one of the things that data insights can help organizations understand as well.
Michael Garel: When we first launched, it was just kind of a basic dashboard. And now we've added all kinds of insights around comparisons, benchmarking,Vulnerabilities, cybersecurity. Where we want to go, where we're looking to take this outside of, there's a whole realm of parts and materials that we can go to. So we're working our on cleaning up all our parts and materials information to be able to provide a lot more insight, being able to create predictive parts ordering so we can tell you when you might need parts in the future based upon past work order history so you can make sure that you have those parts on hand just in time, but you're not sitting on a huge inventory of parts.
Michael Garel: We're looking at different ways that we can apply machine learning, AI, maybe even chatbots, into helping organizations be more efficient in their repair. For example, you might have a device, you have a failure, you're having trouble diagnosing it. It might not have been something that you've ever seen before, but chances are this failure has happened before. And so we can mine all our data and build tools to help you better diagnose that problem and fix it in a much faster fashion. So those are the things that we're looking at.
Michael Garel: We're really looking at making this tool super valuable to not only clinical engineering but also your capital planning folks, your finance folks, your risk folks, patient health and safety, patient quality. So we think that just by being able to mind the CMMS data, we can influence behaviors across all those lines of business.
Michael Garel: We are working on some integrations with Attainia. We've felt with us, the Attania leadership team and customers both, clinical as well as the procurement and planning side, have found value in bringing not only just data insights and Attania together, but also Attainia and the rest of our CMMS platforms as well. So there's a good tie in that whole capital planning, capital purchasing cycle where we can really add some value. So just really excited to engage with a new customer base as well, the equipment planners, and purchasing folks as well. And then having them be able to use our data to help guide their capital plans.
Al Gresch: Awesome.
Mike Zimmer: Well Mike, thank you so much for the time.
Michael Garel: No problem.
Michael Garel: It's always a pleasure talking to you.
Mike Zimmer: I appreciate that, Mike. And to everybody listening, thanks again for taking the time out of your day to learn about the various topics that we cover. Feel free to suggest new topics. Give us your feedback.
Mike Zimmer: That's it for us. We hope you have a great rest of your day.
Al Gresch: Thank you.
Michael Garel: Thank you.
Stay tuned for more episodes from the Healthcare Chats podcast. Submit your questions online and let us know what topics you'd like us to cover.
Feedback is hugely important to us because we rely on followers to plan our next episodes and topics. Leave your suggestions and feedback here.
Your Accruent Healthcare Team
You might also like
Discover what steps hospitals can take to handle the pandemic's many challenges, including a new COVID-19 part predictor for ventilators and ...
Discover how your healthcare organization can save time and money by offloading the administrative burden of parts procurement from your maintenance ...
Hear key takeaways from the 2019 MDExpo Baltimore conference including big data, service management, and capital life cycle management trends.
Subscribe to stay up to date with our latest news, resources and best practices